Study of Adequacy of Commercial Truck Parking Facilities
PDF files can be viewed with the Acrobat® Reader®
2.0 COMMERCIAL TRUCK PARKING DEMAND
2.1 Factors Contributing to Parking Space Demand
This section summarizes an analysis that was conducted to develop an estimate of the peak hour demand for commercial truck parking resulting from the need for drivers to rest and to comply with Federal HOS rules. The two most important factors that contribute to the demand for truck parking are the need to comply with Federal HOS rules and the need for drivers to perform certain non-driving activities (e.g., eating, fueling).
Under the current HOS rules, truck drivers participating in interstate commerce are generally permitted to drive up to 10 hours after 8 consecutive hours off duty. A driver is permitted to be on duty up to 15 hours a day, with 10 hours driving and 5 hours performing non-driving tasks, after which the driver must take off 8 consecutive hours. The regulations further require that, if a motor carrier does not operate commercial vehicles every day of the week, then its drivers may not drive more than 60 hours over a 7‑day period. If the motor carrier does operate commercial vehicles every day of the week, then its drivers may not drive more than 70 hours over an 8-day period. At the end of each time period, drivers are required to take a 24-hour rest break, after which the “HOS clock” restarts. These regulations induce a demand for parking spaces so that drivers who must drive more than 10 hours between their origin and destination (i.e., long-haul drivers) can obtain the required 8 hours of long-term rest. In other words, these rules typically require drivers of commercial motor vehicles to complete a period of rest while en route to a destination if the driver is unable to return home for the required rest.
In addition to the breaks required for achieving long-term rest, drivers also take regular short breaks for activities such as eating, refueling, or using bathroom facilities. These breaks induce a demand for short-term parking spaces at locations that provide amenities to support these activities. While drivers are required to obtain extended rest, there is no single agency, organization, or group that is responsible for providing drivers extended rest locations. Essentially, drivers find such locations themselves and typically rely upon two primary options: commercial truck stops and travel plazas or public rest areas. Commercial truck stops and travel plazas are designed to provide drivers an opportunity to fulfill many non-rest-related activities, while public rest areas provide the driver with only minimal services.
The demand for truck parking along a particular stretch of highway is driven not only by the general factors that induce demand, but also by other factors that affect the distribution of that demand. For example, truck drivers’ desire to accommodate their natural sleep cycles results in greater demand for truck parking spaces at night than during the day. Tight delivery schedules associated with just-in-time delivery can result in demand for truck parking spaces near loading/unloading facilities because drivers use these spaces as staging areas to help ensure on-time delivery. Truckers who drive as teams are likely to have different parking requirements because one team member can drive while the other rests. Also, some States limit parking at public rest areas, encouraging commercial drivers to seek other locations for parkingp>
Taken together, these factors can result in complex demand patterns for truck parking. For example, HOS rules require rest periods away from home primarily for long-haul drivers; a short-haul driver will typically arrive at the destination before a mandatory rest is required. Therefore, highways with a larger proportion of long-haul drivers (relative to the total number of trucks on the road) will typically generate a larger demand for truck parking than other highways. Because short-haul drivers are not required to take an extended rest, one might expect them to take more frequent, shorter breaks, which would favor the use of public rest areas over commercial truck stops and travel plazas. Stretches of a highway that are 8 to 10 hours from a center of commercial traffic might be expected to have higher parking demand because the HOS rules will force drivers from that origin to take an extended rest before driving further. Alternately, an area near a significant commercial vehicle destination may have a substantial early morning parking demand as drivers use rest facilities as staging areas while waiting for the loading/unloading facilities to open.
While the factors listed above help determine the total demand for truck parking in an area (i.e., the latent demand), other factors help determine how that demand is distributed among the available parking locations (i.e., the demand choice). For example, drivers wanting to take a short break are more likely to choose a location for its convenience, while drivers taking a long break are more likely to choose a location that has more favorable amenities. Drivers taking a break for a specific activity (e.g., to take a shower) will park at only a location that supports that activity. If one stretch of highway has a shortage of parking locations, demand that cannot be met on that stretch of highway will be met by parking locations on nearby stretches of highway.
The primary purpose of this section is to discuss the factors that can affect latent demand for commercial vehicle parking and to describe a model to estimate this demand; this helps meet the stated objective of the Section 4027 study to “analyze where shortages exist or are projected to exist.” A secondary goal is to evaluate some of those factors that affect the demand choice because, even in an area in which sufficient parking spaces exist, drivers may still choose to park at inappropriate locations such as on the shoulder of a road. A three-step approach was used to achieve these goals:
- Administer a national survey of driver parking needs and preferences.
- Develop a model for commercial vehicle parking demand.
- Use the model to generate national demand estimates.
2.2 National Survey of Driver Parking Needs and Preferences
The first step in evaluating the demand for commercial vehicle parking was to administer and evaluate a national driver survey about driver parking needs and the adequacy of current parking facilities. This survey accomplished two goals. First, it helped better characterize the factors that affect parking demand, which assisted in the design of the demand model. For example, the survey results helped explain the preference for parking at either a public rest area or a commercial truck stop or travel plaza. Second, the survey provided data that were used to verify some of the assumptions in the model. It has already been noted that the ratio of long-haul to short-haul vehicles could have an important effect on parking demand; the survey results helped identify differences in the parking patterns of long-haul and short-haul drivers. The survey methodology and some of the results of this survey are summarized in this document. A separate report presents a complete description of the national driver survey report.
2.2.1 Survey Methodology
The six-page driver survey was developed with input from a broad spectrum of public- and private-sector stakeholders. The surveys were tested and found to be acceptable during a small pilot or trial survey of 40 respondents at public rest areas and commercial truck stops and travel plazas. The respondents did suggest that distribution at commercial truck stops would be more effective because the quick stops taken by most drivers at public rest areas would not allow time for the completion of the survey.After careful consideration, the advice to survey at only commercial truck stops was followed. To determine whether omitting public rest areas from the list of distribution locations would limit the sample of short-haul drivers, the survey team asked short-haul drivers if they use commercial truck stops and travel plazas as often as they use public rest areas. Short-haul drivers consistently indicated that they use both types of facilities equally. Therefore, to maximize response rate and minimize negative impact on drivers’ time, commercial truck stops and travel plazas were used exclusively for the survey distribution. A geographically stratified sample of commercial truck stops and travel plazas was selected at which to gather data to help ensure a nationally representative distribution of respondents (see figure 1).
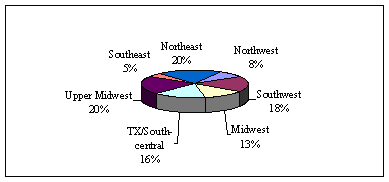 |
Figure 1. Respondent distribution by region.
|
Two methods were used to gather survey results at the selected commercial truck stops and travel plazas. At 20 locations, researchers made site visits and distributed surveys to truck drivers at those sites. The response rate at those sites was 80 percent, collecting a total of 1,042 completed surveys. At 22 other locations, commercial truck stop and travel plaza operators agreed to make the surveys available in heavily traveled areas of the truck stop and to ship back completed surveys. This resulted in nearly 1,100 additional completed surveys.
The charts in Figure 2 describe the distributions of the respondents in terms of the following four factors: (1) long-haul versus short-haul drivers; (2) the size of the company for which the driver works (independent, small, medium, or large); (3) whether the driver often works as part of a driving team (non-teaming, sometimes, or teaming); and (4) the truck volume of the location at which the survey was taken (< 5,000 trucks per day, 5,000 to 15,000 trucks per day, or > 15,000 trucks per day). The distribution of the respondents between long-haul and short-haul drivers is not characteristic of the commercial vehicle driver population; intercept surveys performed at weigh stations indicated that 35 to 65 percent of drivers, depending on the location, are on short-haul trips. This discrepancy between the real-world and sample demographics indicates that care should be taken when interpreting the survey results on factors that may differ between short-haul and long-haul truck drivers. (The survey results primarily represent only long-haul drivers; however, as previously mentioned, a trial survey of short-haul drivers indicated that these drivers use commercial truck stops as often as they use public rest areas. Also, the lack of short-haul driver representation in the sample is due largely to self-selection. When approached by members of the survey team, these drivers indicated that the Truck Parking Needs and Preferences survey was not relevant to them.Most of the short-haul drivers that were approached elected not to participate in the survey.) No attempt was made to confirm whether the other demographic factors are consistent with the population of truck drivers as a whole.
2.2.2 Survey Results
This survey seeks to answer two questions that are fundamental to the truck parking shortage:
- Where do drivers prefer to park, and what factors affect a driver’s decision on where to park?
- Are there sufficient parking spaces available, and if not, what can be done to eliminate the shortages?
Figure 3 addresses the first question, indicating that drivers stopping for a quick nap (two hours or less) have a slight preference for parking at a public rest area, and drivers stopping for an extended rest (more than two hours) strongly prefer a commercial truck stop or travel plaza to a public rest area.
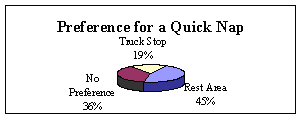 |
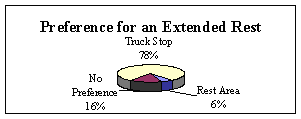 |
Figure 3. Preferred parking locations.
|
The survey addressed parking location by asking drivers where they had most recently stopped for sleep and where they expected to next park their trucks to sleep. Table 1,summarizes the responses to this question and helps validate the results from the previous question; the stated preference for parking at commercial truck stops and travel plazas for sleep is consistent with the locations at which the drivers last parked and expect to park next.
Table 1. Driver parking locations.
Facility
|
Last Stop
|
Next Stop
|
Home
|
9%
|
8%
|
Truck Stop
|
56%
|
58%
|
Public Rest Area
|
8%
|
7%
|
Loading Dock
|
10%
|
14%
|
Ramp
|
4%
|
2%
|
Other
|
11%
|
9%
|
No Response
|
4%
|
4%
|
The second part of the question regarding the preference for truck parking locations is designed to identify the factors that affect a driver’s decision on where to park. One factor is already apparent: the preferred parking location is dependent on whether the driver is making a long or a short stop. Other factors that drivers rate as important when choosing a place to park include availability of restrooms, convenience to the highway, availability of showers, availability of a restaurant, availability of public phones, a well-lit parking area, and availability of fuel. Because of the safety issues involved with parking on ramps and shoulders, the survey specifically asked for the four most common reasons why truck drivers sometimes park on entrance or exit ramps and highway shoulders. The following list includes the most common responses to this question, reinforcing the fact that the dominant problem in truck parking is one of availability; truck drivers do not prefer to park on ramps and shoulders but do park there when unavailable parking elsewhere forces them to.
- No empty spaces are available at nearby facilities (94 percent).
- There are no nearby parking facilities (83 percent).
- Time limits at nearby facilities are too short (50 percent).
- Empty spaces are available at nearby facilities, but access is blocked (50 percent).
The second question, whether a parking shortage exists and how to correct any shortage that does exist, was also addressed in this survey. Figure 4 indicates truck driver opinions on how often parking is available at public rest areas and commercial truck stops and travel plazas.
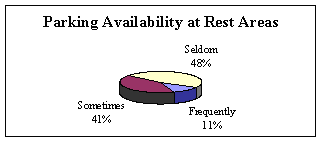 |
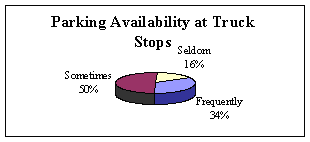 |
Figure 4. Parking availability at public rest areas and commercial truck stops and travel plazas.
|
Truck drivers perceive a significant problem with finding parking at public rest areas and a much smaller problem with finding parking at commercial truck stops and travel plazas. When asked to rank methods for improving truck parking facilities, the respondents identified the following methods as most important:
- Build more commercial truck stop and travel plaza parking spaces – 79 percent listed in top 5.
- Build more public rest area parking spaces – 66 percent listed in top 5.
- Stop enforcement officers from waking driver – 57 percent listed in top 5.
- Eliminate time limits – 49 percent listed in top 5
- Improve parking configuration (e.g., more pull-through parking) – 46 percent listed in top 5.
- Separate truck, car, and recreational vehicle parking – 41 percent listed in top 5.
2.3 National Commercial Vehicle Parking Demand Model
A modeling approach was employed to develop an estimate of the demand for commercial vehicle parking spaces at public facilities (i.e., rest areas, weigh stations) and commercial facilities (e.g., truck stops and travel plazas, motels, fast food restaurants). First, a model was developed to estimate the demand for truck parking for highway segments; this model is summarized in section. Second, field measurements of truck parking demand were made to calibrate the model. The field measurements included all available parking along a highway corridor, including space available at public rest areas, commercial truck stops and travel plazas, hotels and motels, fast food restaurants, shopping malls, and exit ramps. Thus, the calibrated model estimates total demand for parking along a highway corridor. Intermediate model results and model parameters were provided to State partnerships so that they could review the results and model parameters to ensure that they were consistent with local observations. Finally, the model was calibrated and used to estimate the demand for truck parking for all highway segments that are part of the NHS. A complete description of the development and use of the demand model is documented in a separate report, and the following three sections summarize that description.(12)
2.3.1Model Development
This description of the model occurs in two parts. The first part describes a highly simplified version of the model that still captures most of the key elements. This section should be used to become familiar with the general nature and limitations of the model and concludes with a list of factors that are not considered in the simplified model. The second part describes the additional factors that are considered in the final model.
2.3.1.1 The Simplified Demand Model
The simplified model predicts the demand (D) for commercial truck parking spaces along a highway segment based on total truck-hours of travel per day (THT) on that segment and the average parking time per truck-hour of travel (Pavg).
The average truck-hours of travel per day for a segment is estimated from the formula:
THT = Pt · AADT · L/S |
(2) |
where Pt is the percent of vehicles that consists of commercial trucks, AADT is the annual average daily traffic, L is the length of the segment, and S is the speed limit or average truck speed. The term Pavg is a parameter that is estimated during the calibration step to best fit the calibration data. Although this model is conceptually appealing and simple to understand, it does include several limitations. The list below describes some of these limitations and how the simplified model was adapted to help circumvent these limitations. The demand model used in this study is the result of these modifications.
- The goal of this study is to estimate parking shortages that will occur at peak parking demand. The formula used to estimate THT is for the average truck-hours of travel per day, not the peak. This formula should be adjusted to consider both seasonal and daily variations in the truck volume. The demand model includes a term Fs in the estimate for THT that accounts for the seasonal variation in truck volume, and an Fs value of 1.15 is assumed to apply for all segments. In other words, the seasonal peak truck volume is assumed to be 15 percent greater than the annual average daily truck volume. The demand model uses peak parking factors to account for large demands during certain times of the day.
- As described above, the average parking time per truck-hour of travel varies significantly between long-haul and short-haul trucks. The demand model accounts for this difference by estimating the demand separately for long-haul and short-haul trucks and summing these to estimate the total demand. This involves (1) introducing a new term, the short-haul to long-haul ratio (SH/LH), that is used to “split” the total truck-hours of travel per day into the number of truck-hours of travel for short-haul trucks and the number of truck-hours of travel for long-haul trucks and (2) estimating a separate value of Pavg for long-haul and short-haul trucks.
- The term SH/LH can vary dramatically between different highway segments depending on a number of factors, such as the proximity to a population center that is the focus of short-haul transportation and whether the segment is part of a major, long-distance transportation corridor. The demand model uses two values for the term SH/LH: one value is used for segments classified as rural segments, while another value is used for segments classified as urban segments.
- It is important for the model to differentiate between the demand for public parking spaces at public rest areas and private parking spaces at commercial truck stops and travel plazas. The model estimates public and private parking demand by estimating total demand and introducing a factor (PRA), based on drivers’ preferences, representing the percent of total demand that is for public rest area parking and a factor (PTS = 1 - PRA) representing the percent for commercial truck stop and travel plaza parking. The model does not consider variations in these factors that might exist between long-haul and short-haul drivers.
- The parameter Pavg, which represents the ratio of truck parking time at public rest areas and commercial truck stops and travel plazas (including weigh stations, fast food restaurants, hotels, etc.) to the truck driving time, can be estimated directly from survey results that describe the percent of time a truck driver spends driving, at home, and resting away from home. This parameter is influenced by HOS rules, differs between long-haul and short-haul drivers, differs between public and private parking spaces, and may differ with other factors such as geographical region and type of highway. The model includes separate values of Pavg for long-haul and short-haul drivers but does not consider other factors that might affect this parameter. Researchers estimated the value of this parameter based on the limitations imposed by HOS rules and information gathered during the driver survey about how drivers spend their time.
There are other factors that could influence the demand for truck parking (e.g., geographical variations in the short-haul to long-haul ratio). However, the above list summarizes those that were considered in the final demand model developed for this study.
2.3.1.2 The Demand Model
The final demand model uses the general equations and modifications described in the previous section to estimate commercial vehicle parking demand for the NHS. The factors that are used in the final model can be divided into two categories: model variables and model parameters. The model variables (listed in table 2) are the values on the right-hand side of equation (2), which define each highway segment and the commercial vehicle volume on the segment. The values for these variables were obtained from either the Highway Performance Monitoring System[13] (HPMS) or through a State’s own databases and information systems. It is these factors that create differences in the estimates for parking demand for different highway segments.
Table 2. Demand model variables.
Variable
|
Description
|
L
|
Length of the highway segment (mi)
|
AADT
|
Annual average daily traffic (vehicles per day)
|
Pt
|
Percent of daily traffic consisting of commercial trucks
|
S
|
Speed limit of highway, or average truck speed (mi/h)
|
The model parameters are the values (like Fs) that are used to adjust the truck volume estimate of equation (2) and other values that are used to estimate the term Pavg in equation (1). The parameter values were typically estimated from either other data sources, such as the driver surveys, or from calibrating the model based on empirical measurements made as part of this study. Table 3 lists the model parameters and the values of these parameters used in the demand model.
Table 3 Demand model parameters.
Parameter
|
Description
|
Value
|
Fs
|
Seasonal peaking factor
|
1.15
|
SH/LH
|
Short-haul to long-haul ratio
|
0.36/0.64, 0.07/0.93
|
DST
|
Short-term parking duration per hour traveled
|
5 min/h
|
TDRIVING
|
Time driving for long-haul drivers
|
70 h/8 days
|
THOME
|
Time at home for long-haul drivers
|
42 h/8 days
|
TLOAD/UNLOAD
|
Time loading and unloading for long-haul drivers
|
15 h/8 days
|
TSHIPPER/RECEIVER
|
Time at shipper/receiver for long-haul drivers
|
16 h/8 days
|
PRA, PTS
|
Portion of demand for public rest areas/commercial truck stops
|
0.23, 0.77
|
PPFSH
|
Peak-parking factor for short-haul trucks
|
0.02
|
PPFLH
|
Peak-parking factor for long-haul trucks
|
0.09
|
PRLH
|
Long-haul parking ratio
|
0.7833
|
The model parameters are defined as:
- Seasonal peaking factor. This is the ratio of seasonal peak average daily truck volume to average daily truck volume. This value, representing a peak truck volume of 15 percent above the average, was assumed.
- Short-haul to long-haul ratio. This is the ratio of the number of trucks performing short-haul trips to those performing long-haul trips. Because this ratio will be different for segments in populous regions (urban segments) and those in less populous regions (rural segments), two values were used. An origin-destination study performed as part of this study estimated a value of 0.32/0.68 for urban segments. The values of 0.36/0.64 for urban segments and 0.07/0.93 for rural segments were derived during the model calibration. Some States provided specific values for the short-haul to long-haul ratio, in which case those values were used instead of the calibrated values.
- Short-term parking duration per hour traveled. This is the number of minutes (on average) spent parking per hour traveling. A value of five minutes was assumed. This assumption was based on professional judgment and information obtained from talking with drivers about their typical stopping patterns.
- Time driving for long-haul drivers. This is the average number of hours spent driving during an 8-day period. A value of 70 was used, based on HOS rules.
- Time at home for long-haul drivers. This is the average number of hours spent at home during an 8-day period. A value of 42 was derived from responses to the driver survey performed during this study. (On average, drivers stated they sleep at home 6.7 days per month. Multiplying this by 12 months per year, multiplying by 24 hours per day, dividing by 365 days per year, and multiplying by 8 days per 8-day period results in an estimate of 42 hours per 8-day period.)
- Time loading and unloading for long-haul drivers. This is the average number of hours spent loading/unloading during an 8-day period. A value of 15 was derived from responses to the driver survey performed during this study. (The question on which this estimate was based was not part of the original driver survey and was asked of only about 30 drivers.)
- Time at shipper/receiver for long-haul drivers. This is the average hours spent parking at a shipper/receiver (e.g., for rest, waiting to load/unload) during an 8-day period. A value of 16 was derived from responses to the driver survey performed during this study. (On average, drivers stated they stopped for long-term rest at a shipper/receiver 2.6 times per week. Assuming a 6-hour stay each time, drivers spent an average of 16 hours per week resting at these facilities.)
- Portion of demand for public rest areas and commercial truck stops and travel plazas. The proportions of total parking demand for rest area spaces and truck stop spaces were derived from responses to questions in the driver survey regarding where drivers prefer to stop for different activities (e.g., long-term rest, restroom, meal, etc.). The values were derived as follows:1) the number of driver responses for each preference category (i.e., rest area, truck stop, no preference) was weighted according to the average amount of time spent parking for each activity (thereby converting number of drivers into number of truck-hours of parking according to preference); 2) the truck-hours of parking were then summed for each preference category; 3) the truck-hours of parking in the “no preference” category were then divided evenly between the rest-area and truck-stop preference categories; and 4) the total truck-hours of parking for rest areas and truck stops were then divided into the overall total truck-hours of parking. The resulting proportions of parking demand for rest area and truck stop spaces were 0.23 and 0.77, respectively.
- Peak-parking factor for short-haul trucks. This is the ratio of parking demand (in spaces) during peak demand hours to total daily parking demand (in hours) for short-haul trucks. If parking demand was evenly distributed throughout the day, this value would be 1/24 or about 0.04. Because parking demand for short-haul trucks is concentrated during the day and overall peak parking demand occurs at night, this number should be smaller than 0.04. A value of 0.02 was assumed.
- Peak-parking factor for long-haul trucks. This is the ratio of peak parking demand (in spaces) to total daily parking demand (in hours) for long-haul trucks. If parking demand were evenly distributed throughout the day, this value would be 1/24 or about 0.04. Because parking demand for long-haul trucks is concentrated during the overnight hours due to drivers stopping to rest, this number should be greater than 0.04. A value of 0.09 was derived during model calibration.
- Long-haul parking ratio. This is the ratio of the total parking time to the total driving time for long-haul trucks. The following equation is used to estimate this parameter:

2.3.1.3 Model Calibration and Validation
The demand model includes three calibration parameters: the value of SH/LH for rural segments, the value of SH/LH for urban segments, and the value of PPFLH. Table 4 presents the results of the field survey and the final model calibration.
Table 4. Field survey results for model calibration.
Region
|
Corridor
|
Segment
|
Observed Trucks
|
Model Estimate
|
Error
|
Atlanta, GA
|
1
|
I-20 AL State line to Atlanta
|
807
|
550
|
-32%
|
Atlanta, GA
|
1
|
I-75 Atlanta to Macon
|
859
|
1,202
|
40%
|
Atlanta, GA
|
1
|
I-16 Macon to Soperton
|
186
|
158
|
-15%
|
Atlanta, GA
|
1
|
I-16 Soperton to Savannah
|
161
|
194
|
20%
|
Atlanta, GA
|
1
|
Corridor Subtotal
|
2,013
|
2,104
|
4%
|
Atlanta, GA
|
2
|
I-75 Bolingbroke to Cordele
|
641
|
487
|
-24%
|
Atlanta, GA
|
3
|
I-95 Port Wentworth to Darien
|
415
|
473
|
14%
|
Atlanta, GA
|
1-3
|
Region Subtotal
|
3,069
|
3,064
|
0%
|
Pocatello, ID
|
4
|
I-15 UT State line to MT State line1
|
427
|
457
|
7%
|
Pocatello, ID
|
4
|
US-20 Idaho Falls to MT State line1
|
54
|
118
|
119%
|
Pocatello, ID
|
4
|
Corridor Subtotal
|
481
|
575
|
20%
|
Pocatello, ID
|
5
|
I-84 OR State line to Mountain Home 1
|
763
|
530
|
-30%
|
Pocatello, ID
|
5
|
I-84 Mountain Home to UT State line 1
|
817
|
1,003
|
23%
|
Pocatello, ID
|
5
|
I-86 Jct. I-84 to Pocatello 1
|
92
|
174
|
89%
|
Pocatello, ID
|
5
|
Corridor Subtotal
|
1,672
|
1,707
|
2%
|
Pocatello, ID
|
6
|
I-90 WA State line to MT State line 1
|
212
|
206
|
-3%
|
Pocatello, ID
|
6
|
US-12 Lewiston to MT State line 1
|
64
|
83
|
30%
|
Pocatello, ID
|
6
|
Corridor Subtotal
|
276
|
289
|
5%
|
Pocatello, ID
|
4-6
|
Region Subtotal
|
2,429
|
2,571
|
6%
|
Harrisburg, PA
|
7
|
I-81 Jct. I-64 to Harrisonburg 1
|
1,023
|
758
|
-26%
|
Harrisburg, PA
|
7
|
I-81 Harrisonburg to WV State line 1
|
817
|
964
|
18%
|
Harrisburg, PA
|
7
|
I-81 MD State line to Harrisburg 1
|
1,493
|
1,307
|
-12%
|
Harrisburg, PA
|
7
|
I-81 Harrisburg to Frackville 1
|
618
|
1,005
|
63%
|
Harrisburg, PA
|
7
|
|
480
|
574
|
20%
|
Harrisburg, PA
|
7
|
Corridor Subtotal
|
4,431
|
4,608
|
4%
|
Harrisburg, PA
|
8
|
|
654
|
511
|
-22%
|
Harrisburg, PA
|
8
|
|
507
|
383
|
-24%
|
Harrisburg, PA
|
8
|
I-80 Bloomsburg to Scotrun 1
|
546
|
222
|
-59%
|
Harrisburg, PA
|
8
|
Corridor Subtotal
|
1,707
|
1,116
|
-35%
|
Harrisburg, PA
|
7-8
|
Region Subtotal
|
6,138
|
5,724
|
-7%
|
Memphis, TN
|
9
|
I-40 North Little Rock to Brinkley
|
652
|
828
|
27%
|
Memphis, TN
|
9
|
I-40 Wheatley to Memphis
|
808
|
888
|
10%
|
Memphis, TN
|
9
|
I-40 Memphis to Brownsville
|
119
|
373
|
213%
|
Memphis, TN
|
9
|
I-40 Brownsville to Holladay
|
740
|
397
|
-46%
|
Memphis, TN
|
9
|
Corridor Subtotal
|
2,319
|
2,486
|
7%
|
Memphis, TN
|
10
|
I-55 Winona to Batesville
|
322
|
378
|
17%
|
Memphis, TN
|
10
|
I-55 Batesville to Memphis
|
158
|
249
|
58%
|
Memphis, TN
|
10
|
I-55 Memphis to Blytheville
|
934
|
686
|
-27%
|
Memphis, TN
|
10
|
I-55 Holland to Bertrand
|
594
|
536
|
-10%
|
Memphis, TN
|
10
|
Corridor Subtotal
|
2,008
|
1,849
|
-8%
|
Memphis, TN
|
9-10
|
Region Subtotal
|
4,327
|
4,335
|
0%
|
All
|
All
|
TOTAL
|
15,963
|
15,694
|
-2%
|
1Segment classified as a rural segment |
To calibrate the model, field surveys were conducted in four regions to count the number of parked commercial vehicles during the period of peak nighttime parking demand between 10:00 p.m. and 6:00 a.m. Highways in each region were divided into segments, and the potential parking locations along each segment of highway were cataloged during daytime surveys of the highways. Each highway segment was resurveyed during the nighttime period of peak demand. All parked trucks were counted during the nighttime surveys.
The “Segment” column describes the highway segment, and the “Observed Trucks” column lists the results of the field surveys. The “Model Estimate” column lists the estimated demand for truck parking spaces for the calibrated demand model. The “Error” column lists the percentage error in the estimated demand (i.e., “Model Estimate” minus “Observed Trucks” divided by “Observed Trucks”). For example, the demand model underestimates the demand by 32 percent for the first highway segment and overestimates demand by 40 percent for the second.
Travel corridors were also defined based on likely travel routes for commercial vehicles, and the observed and estimated demands for the segments were summed to generate corridor-level values. A corridor subtotal row provides the percentage error in the summed estimated demand for each corridor. Because the observed demand for a highway segment can include unmet demand from nearby segments (e.g., if a highway segment does not have sufficient parking available, some drivers will park on the nearest highway segment with available parking), it was expected that the errors in the corridor-level results would be significantly lower than those at the segment level, which turned out to be true. Note that the error at the segment level does not necessarily indicate that the demand model is inaccurate when applied to highway segments, but may indicate that the lack of available parking spaces on some highway segments creates unmet demand that appears in field observations as unusually high demand on nearby segments with a surplus of parking. Region subtotal rows indicate the estimation error for the cumulative demand for all of the highway segments in the region, and the “-2%” error in the bottom row is for the 29 highway segments combined.
To calibrate the demand model, each highway segment was categorized as either rural or urban.A segment was categorized as urban if it was within 200 miles of a city with a population of 200,000 or more.Otherwise, it was classified as rural. Then, the model variables identified in table 2 were collected for each highway segment, and an iterative process was used to identify the values for the three parameters that enabled the demand model to best estimate the parking demand observed in the field surveys. Table 5 lists the resulting values of the calibration parameters.
The estimates of parking demand corresponding to these model parameters are listed in the “Model Estimate” column of table 4. This table also lists the results for ten highway corridors, four regions, and the total sample defined by combining results for the highway segments. This list indicates that the predictions of the model were more accurate at the corridor and regional level than at the segment level. At the segment level, the average absolute error in estimated demand was 38 percent, while it was 12 percent for the corridors and 3 percent for the regions.
Table 5. Values of calibration parameters.
Parameter
|
Value
|
SH/LH (rural)
|
0.07/0.93
|
SH/LH (urban)
|
0.36/0.64
|
PPFLH
|
0.09
|
While the demand model does provide reasonable estimates of truck parking demand, the following limitations of the model and model calibration were noted:
- The demand model estimates latent demand for each highway segment and ignores variations in the parking supply that might affect the demand actually observed during the field surveys. For example, a driver on a highway section with insufficient parking supply is forced to drive to another segment with sufficient supply. Some of the latent demand for the section with insufficient supply is actually observed on a subsequent section.
- The demand model estimates latent demand caused by typical driver needs to rest and obtain services. It does not consider other factors that might influence demand in a local region, such as the desire to “stage” near a shipper/receiver at night in order to more easily access the loading dock in the morning.
- The fact that the SH/LH ratio varies was reflected in the model by classifying each highway segment as either rural or urban based on its proximity to a large city and using a different value for this parameter for rural and urban segments. This methodology may not capture the true variation in this parameter, and a more realistic model for estimating this ratio for each highway segment would likely result in better demand estimates.
- Demand for parking along half the 29 segments used for calibration was at or exceeding capacity, limiting the number of drivers who could find parking along those segments. Thus, the field surveys may have underestimated the actual demand (by not counting those drivers who wanted to park but could not), which would result in a calibrated model that also underestimates demand.
- Although the model provides more accurate demand estimates for larger geographical regions, evaluations based on large geographic regions may not be an appropriate way to evaluate truck parking shortages—an excess supply at one extreme of a large geographical region does not help a tired driver at the other extreme of the region to find rest.
To examine the validity of the model, the demand estimates generated by the model were compared against observed demand measured by the States of Iowa and South Dakota. Table 6 lists the results of this comparison.Neither of these comparisons is an exact comparison to the demand estimates generated by the demand model. The Iowa study was conducted in 1999 (not 2000), and the observed parking demand included only vehicles parked at public rest areas and at 39 of 58 commercial truck stops located near the Interstate highways. The study excluded vehicles parked at other public locations (e.g., weigh stations) and on exit ramps, and total demand for parking at commercial truck stops was estimated by extrapolating the observed values. In the South Dakota study, it was not clear whether the demand included trucks parked at other public locations (e.g., weigh stations) and on exit ramps. Despite these limitations in the compatibility of these surveys with the demand estimates, the comparison supports the conclusions drawn from the model calibration results listed in table 4.
Table 6. State survey results for model validation.
State
|
Highway
|
Observed Demand
|
Estimated Demand
|
Error
|
|
I-29
|
599
|
392
|
-35%
|
I-35
|
487
|
601
|
23%
|
I-80
|
1,813
|
1,844
|
2%
|
I-380
|
275
|
153
|
-44%
|
Total
|
3,174
|
2,990
|
-6%
|
|
I-29
|
243
|
|
42%
|
I-90
|
532
|
519
|
-2%
|
Total
|
775
|
865
|
12%
|
2.4 National Demand for Commercial Vehicle Parking
The demand model described in the previous section of this document was used to estimate parking demand for corridors along the Interstate and non-Interstate portions of the NHS using procedures outlined in the technical guidance document. (11) The process for developing parking demand estimates requires three steps:
- Identify major trucking corridors and select analysis segments.
- Collect demand model inputs.
- Apply truck parking demand model to estimate peak hour demand.
The explanation of each step is as follows:
- Identify major trucking corridors and select analysis segments. The modeling approach was applied to appropriate NHS roadway segments throughout 49 States (excluding Hawaii). The corridors considered for analysis were identified by examining the amount of commercial vehicle traffic carried by a highway. These corridors generally consist of Interstate highways and non-Interstate highways that carry a significant amount of commercial vehicle traffic, including roadways that carry more than 1,000 combination vehicles per day. Additional NHS roads were considered if they were part of an identified national corridor.
National corridors were selected on the basis of utilization, network continuity, and geographic distribution. As a rule, routes were initially identified on the basis of commodity flows. Rather than relying on strictly numerical criteria for identification of routes, the research team used visual scans of commodity flows on a State-by-State basis, using the relative magnitude of commodity flows as an initial selection approach. On this basis, the routes with the dominant volumes of commercial vehicle activity within each State were chosen.
To ensure network continuity, the study team also looked at cross-border issues. In some instances, routes were selected because they accounted for critical links in regional or trans-regional commodity flows. Consideration was also given to the National Corridor Planning & Development Program and Coordinated Border Infrastructure Program (also known as CORBOR). CORBOR is looking at the 43 high-priority corridors on the NHS and at projects that improve transportation near our borders with Canada and Mexico. The intent of looking at CORBOR was to consider national and international trade implications of the national corridors.
Once the proposed national corridors were identified, the corridors were segmented so that the demand model could be applied to the highway segments. Segments were identified to be consistent with the technical guidance document and with a logical partition of the proposed corridors. Proposed corridors and candidate segments were then presented to the States for comment; in some instances, the States proposed changes either in the routes selected or in the segmentation of the corridors. In other cases, segments were excluded because there were not sufficient parking supply data or a practical method for estimating truck volume. The resulting highway segments are typically between 60 and 120 miles long and carry a uniform amount of commercial vehicle traffic.
- Collect demand model inputs. To apply the demand model, the length, travel speed, annual average daily traffic (AADT), and percent trucks must be known for each segment, and estimates for these parameters were collected from a variety of sources. Although the data sources were generally the same for each State, there were some differences. The data sources typically included the following:
- Length values were estimated by referring to highway maps.
- Speed limit values were based on HPMS and augmented by comments provided by the State DOT.
- AADT and projected AADT were derived from HPMS and augmented by public rest area survey responses and comments provided by the State DOT.
- Some State DOTs provided additional comments and corrections to AADT and other values.
- When other sources of traffic flow data were not available, the “Heavy Commercial Vehicle Flow Atlas of the United States National Highway System” (revised on August 27, 1998) was used.
Partners in each State reviewed these estimates and provided comments and suggestions that were used to improve the estimates. In many cases, adjustments were made to better reflect the volume of truck traffic on a segment, the speed limit, and other model factors. For example, the following States suggested different values for the short-haul to long-haul ratio for some highway segments: Idaho used a 0.20/0.80 ratio; Alaska used a 0.75/0.25 ratio because all the routes in Alaska can be driven in a single day; Kansas, Michigan, and Washington used a 0.60/0.40 ratio for some highly urban highway segments; and South Dakota used a 0.03/0.97 ratio for some highly rural highway segments. After the States completed their review of the model variable and parameter data, a final calibration of the demand model was completed that generated final values for the short-haul to long-haul ratios and the long-haul peak parking factor. The collected data and the calibrated parameters were used for the final demand estimates.
- Apply truck parking demand model to estimate peak hour demand. After defining the highway segments and identifying estimates for the model variables, the demand model was used to estimate the parking demand for each segment. The demand estimates (as well as the demand model inputs) for each section are listed in the Final State Status Reports. The demand estimates for each segment were summed to generate demand estimates for each State ( table 7 ) and for Interstate and non-Interstate highways. In this table, the “Rest Area” and “Truck Stop” columns list the estimated demand for truck parking at public rest area and commercial truck stops and travel plazas, respectively, and the “Total” column lists the estimated total truck parking demand. The total demand on Interstate highways was for 245,389 truck parking spaces (56,424 spaces at public and 188,965 at commercial facilities), and the total demand on non-Interstate highways was for 41,927 spaces (9,643 at public and 32,284 at commercial facilities). The demand estimates are for 39,963 miles of Interstate highway, which cover most of the Interstate highways, and 21,702 miles of non-Interstate highway, which include only those sections of non-Interstate highways with a significant volume of commercial vehicle traffic
The “Annual Increase” column lists the estimated annual increase in truck parking demand for each State over the next 20 years, which is estimated from corresponding estimates for the increase in truck volume over this period. In other words, the same demand model was used to estimate the demand for truck parking in 2000 and in 2020, and the “Annual Increase” was calculated as the annual growth rate necessary to account for the growth in the estimated demand between 2000 and 2020. Note that, because most States could provide only forward projections for AADT values, the other model variables and parameters (e.g., percent trucks, speed limit) were assumed to remain fixed between 2000 and 2020.
While 35 States elected to use these demand model results to satisfy the requirements of the Section 4027 study, 14 States elected to use a different method of evaluating the demand for and supply of truck parking spaces. Table 8 lists the State demand estimates and compares these estimates to those of the demand model.
Table 7. Commercial truck parking demand: Peak hour demand along interstates and other NHS routes carrying more than 1,000 trucks per day, 2000.
State
|
Rest Area Demand
|
Truck Stop Demand
|
Total Demand
|
Estimated Annual Increase in Demand
|
Alabama
|
1,634
|
5,473
|
7,107
|
4.4%
|
Alaska
|
25
|
88
|
113
|
1.0%
|
Arizona
|
1,052
|
3,523
|
4,575
|
3.2%
|
Arkansas
|
1,783
|
5,968
|
7,751
|
2.9%
|
California
|
4,539
|
15,183
|
19,722
|
1.9%
|
Colorado
|
760
|
2,546
|
3,306
|
3.0%
|
Connecticut
|
616
|
2,060
|
2,676
|
1.7%
|
Delaware
|
206
|
694
|
900
|
2.4%
|
Florida
|
1,694
|
5,665
|
7,359
|
2.8%
|
Georgia
|
2,188
|
7,324
|
9,512
|
3.0%
|
Idaho
|
734
|
2,462
|
3,196
|
3.0%
|
Illinois
|
3,338
|
11,172
|
14,510
|
1.1%
|
Indiana
|
4,299
|
14,400
|
18,699
|
3.0%
|
Iowa
|
688
|
2,302
|
2,990
|
3.6%
|
Kansas
|
566
|
1,907
|
2,473
|
2.7%
|
Kentucky
|
2,206
|
7,380
|
9,586
|
2.7%
|
Louisiana
|
2,060
|
6,910
|
8,970
|
3.0%
|
Maine
|
205
|
691
|
896
|
0.5%
|
Maryland
|
592
|
1,983
|
2,575
|
2.0%
|
Massachusetts
|
863
|
2,894
|
3,757
|
1.3%
|
Michigan
|
1,275
|
4,262
|
5,537
|
2.2%
|
Minnesota
|
872
|
2,925
|
3,797
|
2.0%
|
Mississippi
|
1,254
|
4,194
|
5,448
|
2.7%
|
Missouri
|
2,643
|
8,841
|
11,484
|
2.7%
|
Montana
|
462
|
1,550
|
2,012
|
2.6%
|
Nebraska
|
251
|
837
|
1,088
|
3.6%
|
Nevada
|
682
|
2,285
|
2,967
|
2.0%
|
New Hampshire
|
72
|
243
|
315
|
2.2%
|
New Jersey
|
457
|
1,528
|
1,985
|
0.6%
|
New Mexico
|
1,218
|
4,083
|
5,301
|
2.5%
|
New York
|
1,801
|
6,034
|
7,835
|
3.0%
|
North Carolina
|
1,270
|
4,262
|
5,532
|
3.0%
|
North Dakota
|
188
|
635
|
823
|
3.0%
|
Ohio
|
3,301
|
11,059
|
14,360
|
2.9%
|
Oklahoma
|
1,078
|
3,610
|
4,688
|
1.8%
|
Oregon
|
1,139
|
3,819
|
4,958
|
1.8%
|
Pennsylvania
|
2,360
|
7,903
|
10,263
|
3.0%
|
Rhode Island
|
167
|
566
|
733
|
1.4%
|
South Carolina
|
1,265
|
4,236
|
5,501
|
3.8%
|
South Dakota
|
199
|
666
|
865
|
1.7%
|
Tennessee
|
1,214
|
4,073
|
5,287
|
4.0%
|
Texas
|
8,305
|
27,797
|
36,102
|
2.7%
|
Utah
|
391
|
1,307
|
1,698
|
4.3%
|
Vermont
|
27
|
91
|
118
|
1.2%
|
Virginia
|
1,772
|
5,932
|
7,704
|
1.4%
|
Washington
|
815
|
2,724
|
3,539
|
2.1%
|
West Virginia
|
468
|
1,572
|
2,040
|
3.0%
|
Wisconsin
|
633
|
2,115
|
2,748
|
4.2%
|
Wyoming
|
440
|
1,475
|
1,915
|
3.6%
|
TOTAL
|
66,067
|
221,249
|
287,316
|
2.7%
|
Table 8. Commercial truck parking demand: Comparison of state and demand model estimates.
State
|
Model
|
Public Rest Area Demand
|
Private Truck Stop Demand
|
Total Demand
|
Alaska
|
State Estimate1 Demand Model
|
68
25
|
97
88
|
165
113
|
California
|
State Estimate2 Demand Model
|
9,162
4,539
|
13,595
15,183
|
22,757
19,722
|
Colorado
|
State Estimate2 Demand Model
|
1,491
760
|
2,212
2,546
|
3,703
3,306
|
Connecticut
|
State Estimate3 Demand Model
|
1,462
616
|
2,060
|
2,676
|
Idaho
|
State Estimate4 Demand Model
|
567
734
|
1,886
2,462
|
2,453
3,196
|
Maine
|
State Estimate5 Demand Model
|
205
205
|
691
691
|
896
896
|
Nebraska
|
State Estimate2 Demand Model
|
519
251
|
769
837
|
1,288
1,088
|
Ohio
|
State Estimate2 Demand Model
|
6,931
3,301
|
10,294
11,059
|
17,225
14,360
|
Rhode Island
|
State Estimate6 Demand Model
|
228
167
|
766
566
|
994
733
|
South Dakota
|
State Estimate7 Demand Model
|
179
199
|
905
666
|
1,084
865
|
Tennessee
|
State Estimate 8 Demand Model
|
1,214
|
4,073
|
5,287
|
Vermont
|
State Estimate 9 Demand Model
|
27
|
91
|
118
|
West Virginia
|
State Estimate 10 Demand Model
|
468
|
1,572
|
2,040
|
Wyoming
|
State Estimate11 Demand Model
|
440
|
1,475
|
1,915
|
1Used a preliminary version of the demand model, which is the same as the final model but with the following different parameter values: Short-haul to Long-haul (SH-LH) Ratio = 0.40/0.60, Long-haul Parking Ratio = 1.25, Long-haul Peak Parking Factor (PPF) = 0.07, Short-haul Percent Public/Private = 0.6/0.4, and Long-haul Percent Public/Private = 0.4/0.6. Alaska customized the preliminary model by using a SH-LH Ratio of 0.75/0.25 for all highway segments.(11) 2Used a preliminary version of the demand model, which is the same as the final model but with the following different parameter values: SH-LH Ratio = 0.40/0.60, Long-haul Parking Ratio = 1.25, Long-haul PPF = 0.07, Short-haul Percent Public/Private = 0.6/0.4, and Long-haul Percent Public/Private = 0.4/0.6.(11) 3Used the model from a 1996 study.(1) This model estimates demand at a public rest stop based primarily on the annual average daily traffic of the road served by the rest stop.
4Used a version of the demand model, which is the same as the final model but with the following different parameter values: SH-LH Ratio = 0.20/0.80 and Long-haul PPF = 0.08.
5Maine has hired a consultant to perform the required rest area study, and the results of that study are not yet available. In the interim, Maine has accepted preliminary numbers generated using the demand model.
6Did not specify the model used to generate the State estimates.
7Used a preliminary version of the demand model, which is the same as the final model but with the following different parameter values: SH-LH Ratio = 0.40/0.60, Long-haul Parking Ratio = 1.25, Long-haul PPF = 0.07, Short-haul Percent Public/Private = 0.6/0.4, and Long-haul Percent Public/Private = 0.4/0.6. South Dakota customized the preliminary model by using different SH-LH Ratios (0.03/0.97, 0.1/0.9, and 0.4/0.6) on different highway segments.(11) South Dakota also conducted field surveys to validate the model predictions.
8Used a model proposed in 1981 by the Federal Highway Administration in the report Safety Rest Area: Planning, Location, Design, which estimates demand at public rest areas based primarily on traffic counts of vehicles entering the rest areas. Tennessee also conducted field studies to help evaluate usage of public rest areas.
9Vermont conducted a field survey of rest area usage and did not estimate parking demand.
10West Virginia did not provide estimates of rest area demand.
11Wyoming inventoried the supply of parking spaces along highway segments and evaluated the adequacy of that supply by comparing it to the daily count of trucks using each highway segment. Wyoming used driver interviews to generate demand estimates for a highway segment only when this analysis indicated a potential supply inadequacy.
|
Previous | Table of Contents | Next
|